HDFS Design Principles
Design Goals
Store very large files: “very large” here means several hundred M, G, or even TB.
Adopt a stream-based data access method: HDFS is based on the assumption that the most effective data processing mode is to generate or copy a data set once and then do a lot of analysis work on it. Analysis work often reads most of the data in the data set, even if not all of it. Therefore, the time required to read the entire data set is more important than the delay in reading the first record.
Run on commercial hardware: Hadoop does not require special expensive, reliable machines and can run on ordinary commercial machines (which can be purchased from multiple vendors). Commercial machines do not mean low-end machines. In a cluster (especially a large one), the node failure rate is relatively high. HDFS’s goal is to ensure that the cluster does not cause significant interruptions to users when nodes fail.
Application Types Not Suitable for HDFS
Some scenarios are not suitable for storing data in HDFS. Here are a few examples:
Low-latency data access
Applications that require latency in the millisecond range are not suitable for HDFS. HDFS is designed for high-throughput data transmission, so latency may be sacrificed. HBase is more suitable for low-latency data access.
A large number of small files
The metadata of files (such as directory structure, node list of file blocks, and block-node mapping) is stored in the memory of the NameNode. The number of files in the entire file system is limited by the memory size of the NameNode. As a rule of thumb, a file/directory/file block generally occupies 150 bytes of metadata memory space. If there are one million files, each file occupies one file block, which requires about 300M of memory. Therefore, the number of files in the billions is difficult to support on existing commercial machines.
Multiple reads and writes, requiring arbitrary file modification
HDFS writes data in an append-only manner. It does not support arbitrary offset modification of files. It does not support multiple writers.
HDFS Positioning
To improve scalability, HDFS uses a master/slave architecture to build a distributed storage cluster, which makes it easy to add or remove slaves to the cluster.
HDFS is an important component of the Hadoop ecosystem. It is a distributed file system designed to store large amounts of data and provide high-throughput data access. HDFS is designed to store data on inexpensive hardware and provide high fault tolerance. It achieves this goal by distributing data to multiple nodes in the cluster. HDFS is positioned as a batch processing system suitable for offline processing of large-scale data.
The main features of HDFS include:
- High fault tolerance: HDFS distributes data to multiple nodes, so even if a node fails, data can still be accessed through other nodes.
- High throughput: HDFS is designed to support batch processing of large-scale data, so it provides high-throughput data access.
- Suitable for large files: HDFS is suitable for storing large files because it divides files into multiple blocks for storage and distributes these blocks to multiple nodes.
- Stream data access: HDFS supports stream data access, which means it can efficiently process large amounts of data streams.
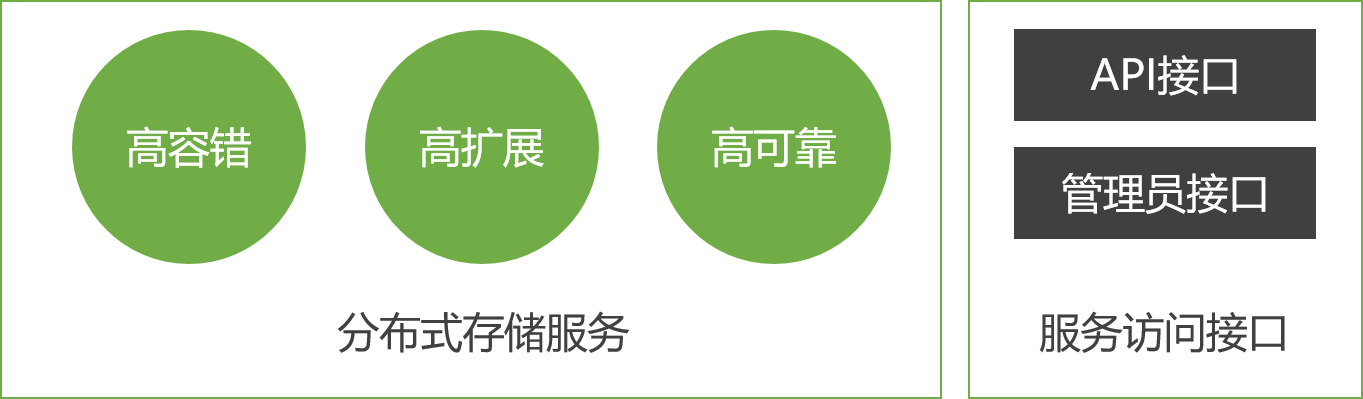
HDFS Architecture
HDFS uses a master/slave architecture to build a distributed storage service, which improves the scalability of HDFS and simplifies the architecture design. HDFS stores files in blocks, optimizing storage granularity. The NameNode manages the storage space of all slave machines, while the DataNode is responsible for actual data storage and read/write operations.
Blocks
There is a concept of blocks in physical disks. The physical block of a disk is the smallest unit of disk operation for reading and writing, usually 512 bytes. The file system abstracts another layer of concepts on top of the physical block of the disk, and the file system block is an integer multiple of the physical disk block. Generally, it is several KB. The blocks in Hadoop are much larger than those in general single-machine file systems, with a default size of 128M. The file in HDFS is split into block-sized chunks for storage, and these chunks are scattered across multiple nodes. If the size of a file is smaller than the block size, the file will not occupy the entire block, only the actual size. For example, if a file is 1M in size, it will only occupy 1M of space in HDFS, not 128M.
Why are HDFS blocks so large?
To minimize the seek time and control the ratio of time spent locating and transmitting files. Assuming that the time required to locate a block is 10ms and the disk transmission speed is 100M/s. If the proportion of time spent locating a block to the transmission time is controlled to 1%, the block size needs to be about 100M. However, if the block is set too large, in MapReduce tasks, if the number of Map or Reduce tasks is less than the number of cluster machines, the job efficiency will be very low.
Benefits of block abstraction
- The splitting of blocks allows a single file size to be larger than the capacity of the entire disk, and the blocks that make up the file can be distributed across the entire cluster. In theory, a single file can occupy the disk of all machines in the cluster.
- Block abstraction also simplifies the storage system, without worrying about its permissions, owner, and other content (these contents are controlled at the file level).
- Blocks are the unit of replication in fault tolerance and high availability mechanisms.
Namenode & Datanode
The entire HDFS cluster consists of a master-slave model of Namenode and Datanode. The Namenode stores the file system tree and metadata of all files and directories. The metadata is persisted in two forms:
However, the persistent data does not include the node list where the block is located and which nodes the file blocks are distributed to in the cluster. This information is reconstructed when the system is restarted (through the block information reported by the Datanode). In HDFS, the Namenode may become a single point of failure for the cluster. When the Namenode is unavailable, the entire file system is unavailable. HDFS provides two solutions to single point of failure:
Backup persistent metadata
Write the file system metadata to multiple file systems at the same time, such as writing metadata to both the local file system and NFS at the same time. These backup operations are synchronous and atomic.
Secondary Namenode
The Secondary node periodically merges the namespace image and edit log of the main Namenode to avoid the edit log being too large, and merges them by creating a checkpoint. It maintains a merged namespace image replica that can be used to recover data when the Namenode completely crashes. The following figure shows the management interface of the Secondary Namenode:
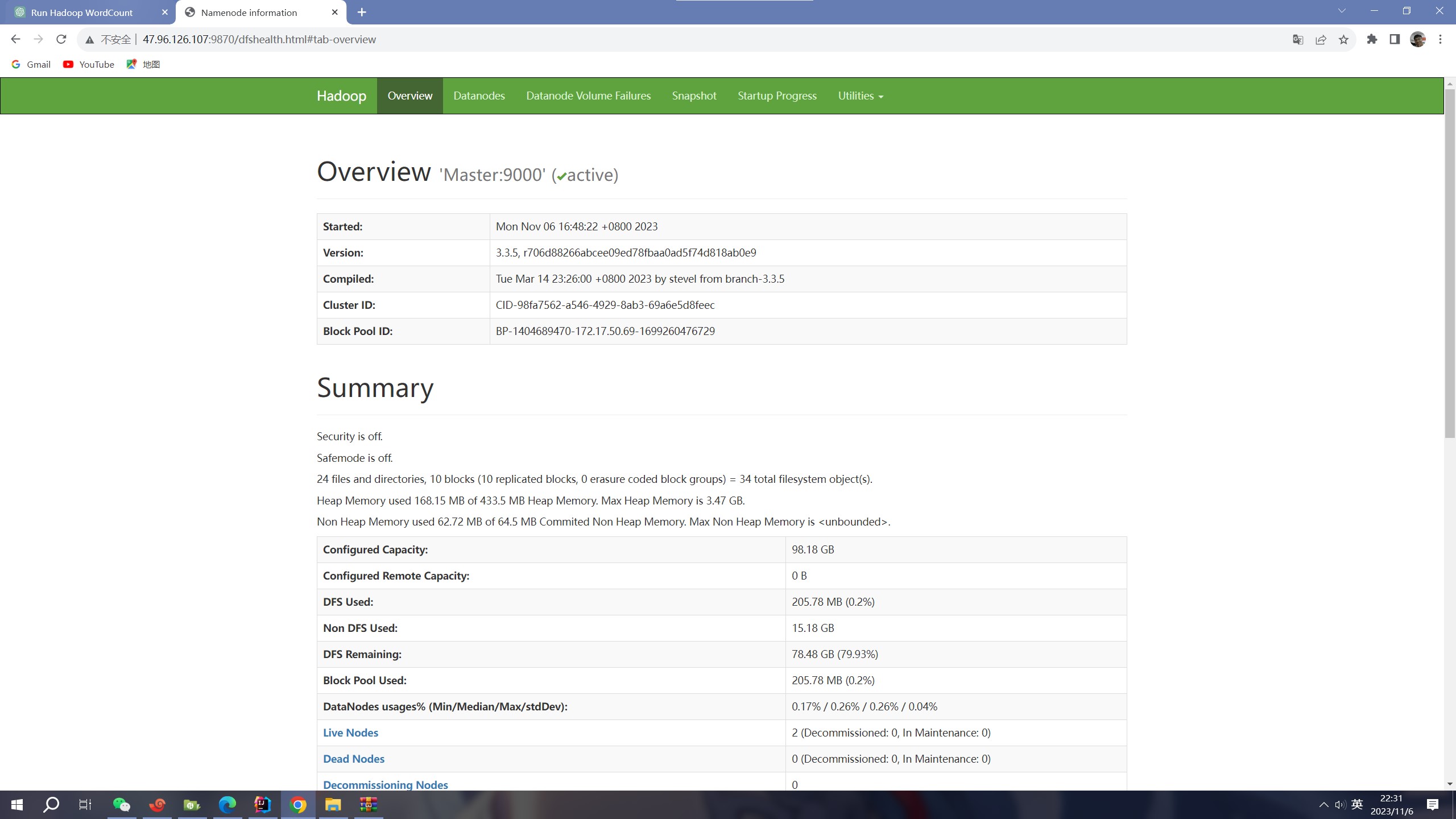
Internal Features of HDFS
Data Redundancy
HDFS stores each file as a series of data blocks, with a default block size of 64MB (configurable).
For fault tolerance, all data blocks of a file have replicas (the replication factor is configurable).
HDFS files are written once and strictly limited to only one write user at any time.
Replica Placement
HDFS clusters usually run on multiple racks, and communication between machines on different racks requires switches.
HDFS uses a rack-aware strategy to improve data reliability, availability, and network bandwidth utilization.
Rack failures are much less common than node failures, and this strategy can prevent data loss when an entire rack fails, improve data reliability and availability, and ensure performance.
Replica Selection
HDFS tries to use the replica closest to the program to meet user requests, reducing total bandwidth consumption and read latency.
The HDFS architecture supports data balancing strategies.
Heartbeat Detection
The NameNode periodically receives heartbeats and block reports from each DataNode in the cluster, indicating that the DataNode is working properly.
The NameNode marks DataNodes that have not sent heartbeats recently as down and does not send them any new I/O requests.
The NameNode continuously checks these data blocks that need to be replicated and re-replicates them when necessary.
Data Integrity Check
- For various reasons, the data block obtained from the DataNode may be corrupted.
Classic HDFS Architecture
The NameNode is responsible for managing the metadata of the file system, while the DataNode is responsible for storing the actual data of the file blocks. This division of labor enables HDFS to efficiently store and manage large-scale data.
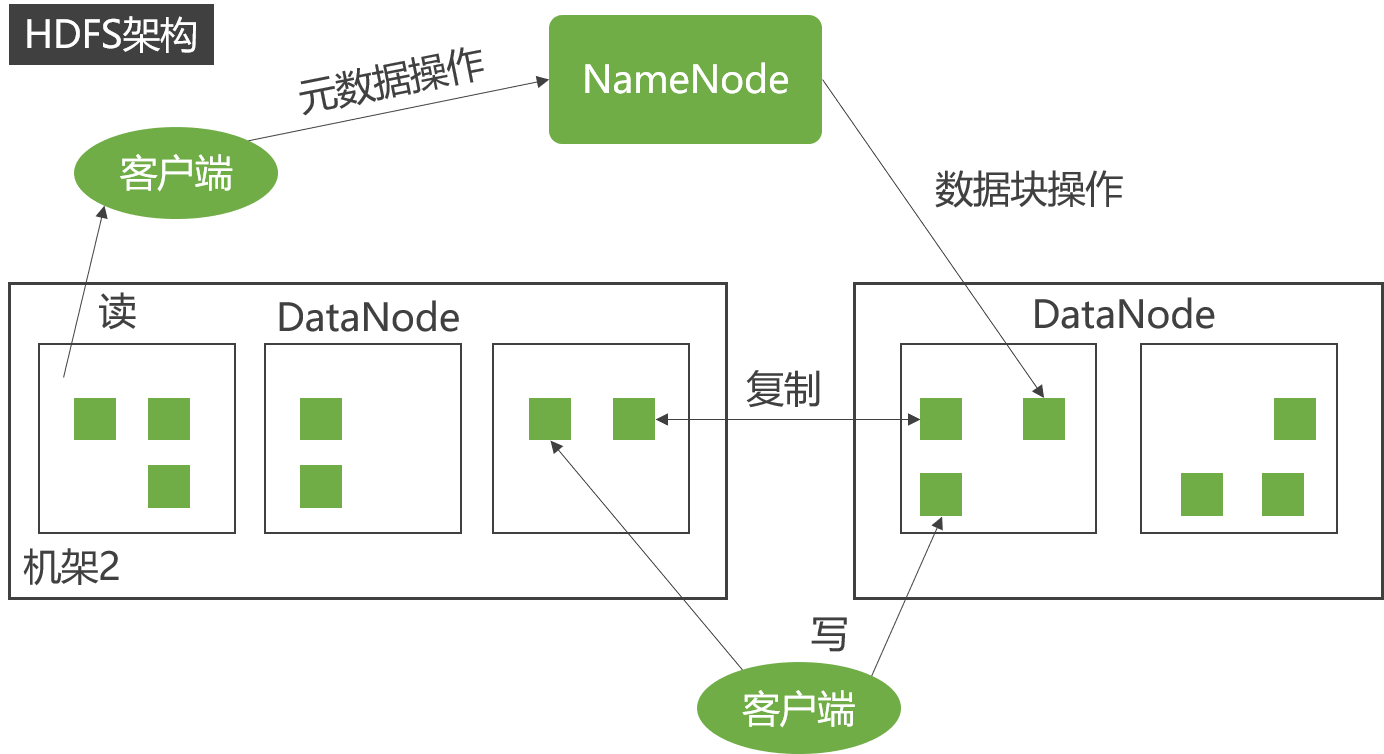
Specifically, when a client needs to read or write a file, it sends a request to the NameNode. The NameNode returns the metadata information of the file and the location information of the file blocks. The client communicates with the DataNode based on this information to read or write the actual data of the file blocks.
Therefore, the NameNode and DataNode play different roles in the HDFS architecture.
What is the difference in function?
HDFS is an abbreviation for Hadoop Distributed File System, an important component of the Hadoop ecosystem. The HDFS architecture includes one NameNode and multiple DataNodes. The NameNode is the master node of HDFS, responsible for managing the namespace of the file system, the metadata information of the file, and the location information of the file blocks. The DataNode is the slave node of HDFS, responsible for storing the actual data of the file blocks.
Specifically, when a client needs to read or write a file, it sends a request to the NameNode. The NameNode returns the metadata information of the file and the location information of the file blocks. The client communicates with the DataNode based on this information to read or write the actual data of the file blocks.
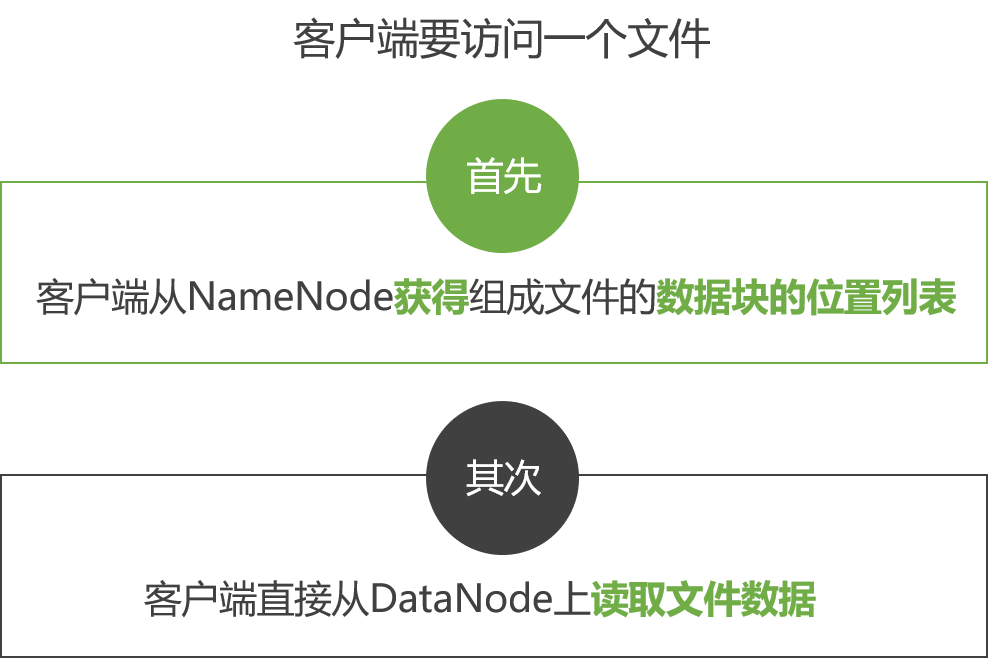
General Topology
There is only one NameNode node, and the SecondaryNameNode or BackupNode node is used to obtain NameNode metadata information in real time and back up metadata.
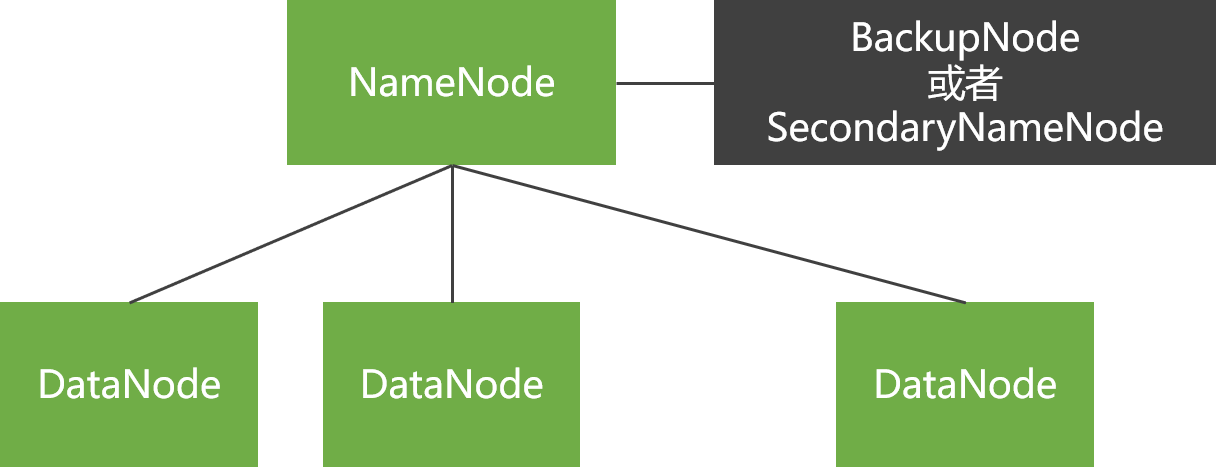
Commercial Topology
There are two NameNode nodes, and ZooKeeper is used to implement hot standby between NameNode nodes.
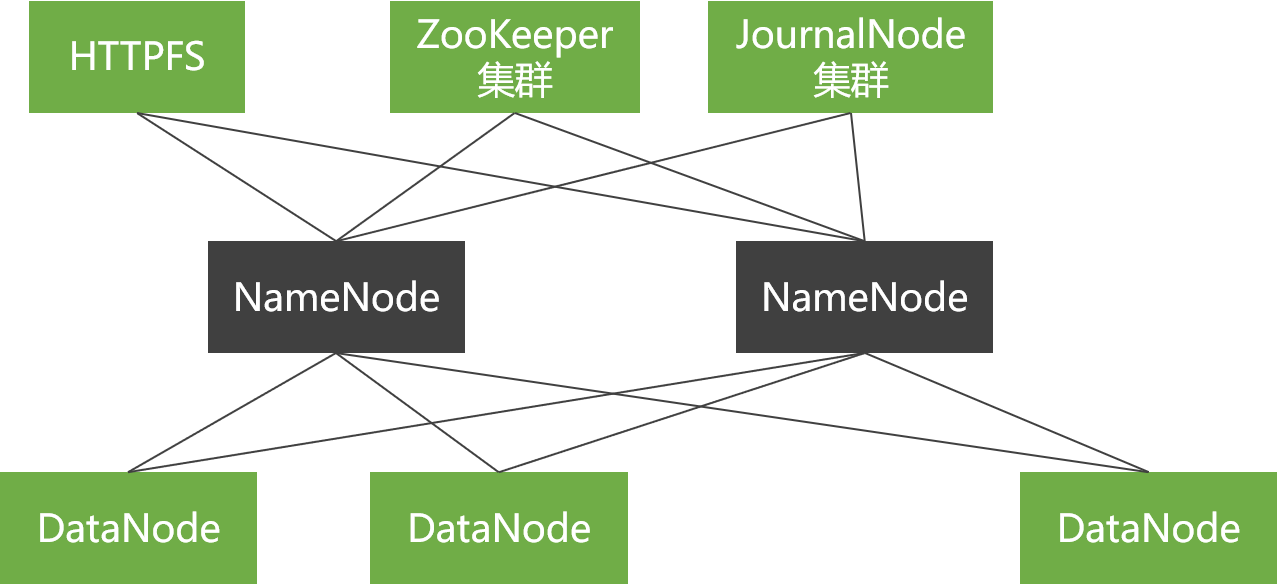
Command Line Interface
HDFS provides various interaction methods, such as Java API, HTTP, and shell command line. Command line interaction is mainly operated through hadoop fs. For example:
hadoop fs -copyFromLocal // Copy files from local to HDFS
hadoop fs mkdir // Create a directory
hadoop fs -ls // List file list
In Hadoop, the permissions of files and directories are similar to the POSIX model, including three permissions: read, write, and execute.
Read permission (r): Used to read files or list the contents of a directory
Write permission (w): For files, it is the write permission of the file. The write permission of the directory refers to the permission to create or delete files (directories) under the directory.
Execute permission (x): Files do not have so-called execute permissions and are ignored. For directories, execute permission is used to access the contents of the directory.
Each file or directory has three attributes: owner, group, and mode:
Owner: Refers to the owner of the file
Group: For permission groups
Mode: Consists of the owner’s permissions, the permissions of the members of the file’s group, and the permissions of non-owners and non-group members.
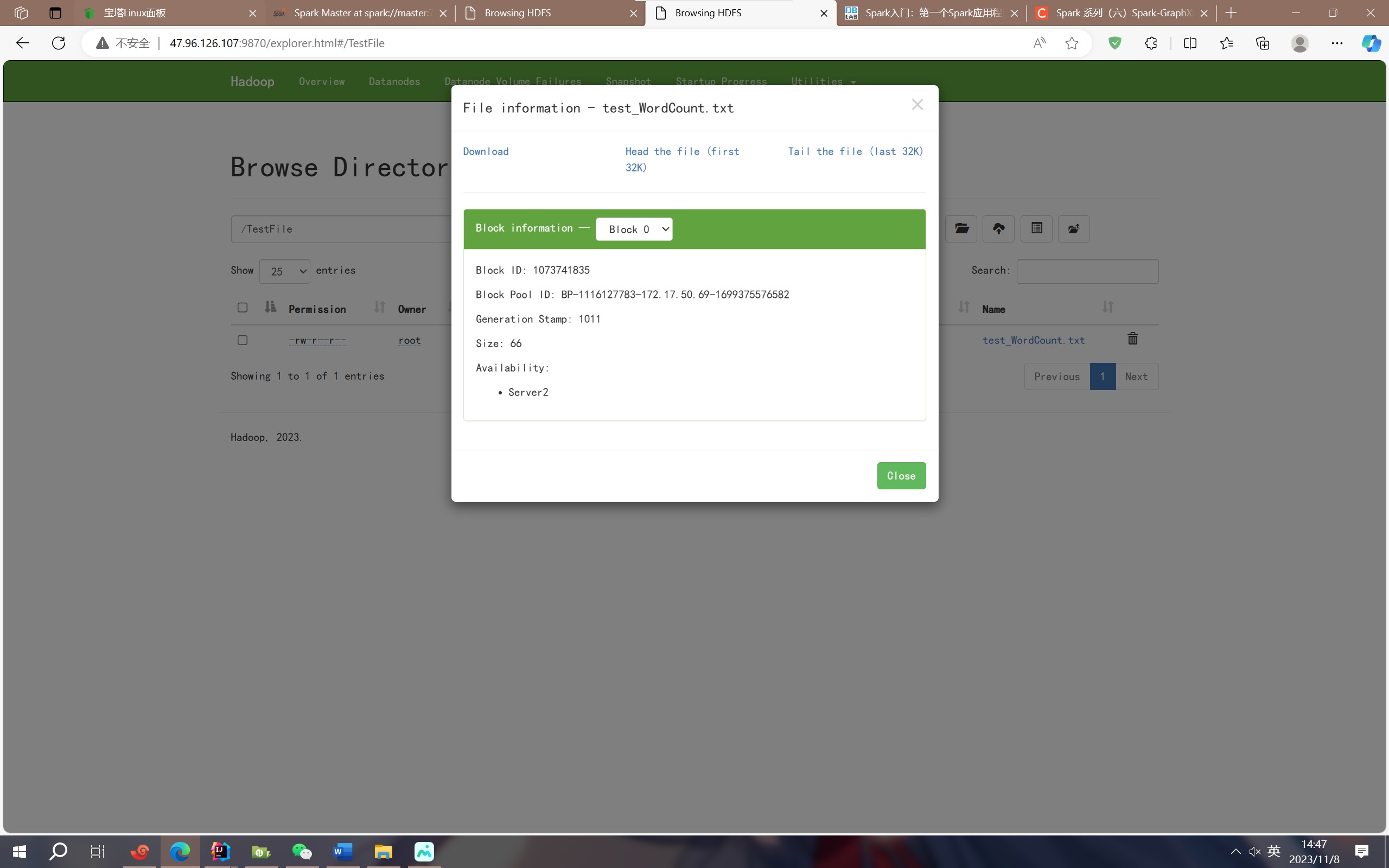
Data Flow (Read and Write Process)
Read File
The rough process of reading a file is as follows:
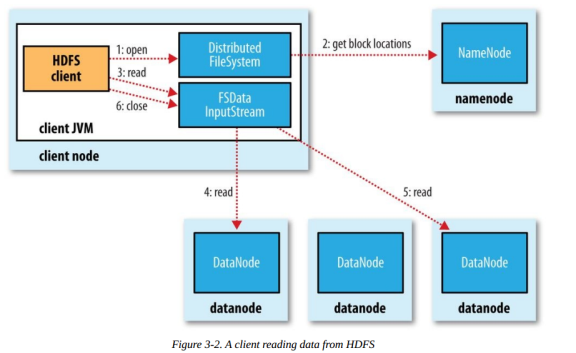
The client passes a file Path to the FileSystem’s open method.
DFS uses RPC to remotely obtain the datanode addresses of the first few blocks of the file. The NameNode determines which nodes to return based on the network topology structure (provided that the node has a block replica). If the client itself is a DataNode and there is a block replica on the node, it is read directly from the local node.
The client uses the FSDataInputStream object returned by the open method to read data (call the read method).
The DFSInputStream (FSDataInputStream implements this class) connects to the node that holds the first block and repeatedly calls the read method to read data.
After the first block is read, find the best datanode for the next block and read the data. If necessary, DFSInputStream will contact the NameNode to obtain the node information of the next batch of Blocks (stored in memory, not persistent), and these addressing processes are invisible to the client.
After the data is read, the client calls the close method to close the stream object.
During the data reading process, if communication with the DataNode fails, the DFSInputStream object will try to read data from the next best node and remember the failed node, and subsequent block reads will not connect to the node.
After reading a Block, DFSInputStram performs checksum verification. If the Block is damaged, it tries to read data from other nodes and reports the damaged block to the NameNode.
Which DataNode does the client connect to get the data block is guided by the NameNode, which can support a large number of concurrent client requests, and the NameNode evenly distributes traffic to the entire cluster as much as possible.
The location information of the Block is stored in the memory of the NameNode, so the corresponding location request is very efficient and will not become a bottleneck.
Write File
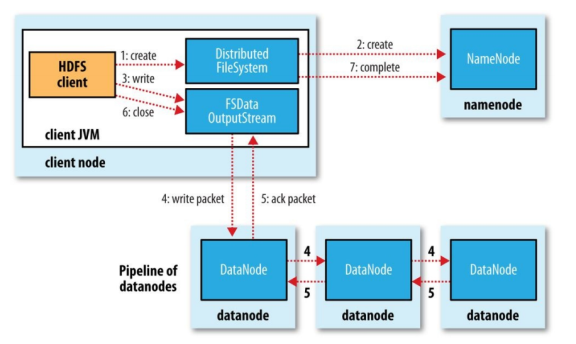
Step breakdown
The client calls the create method of DistributedFileSystem.
DistributedFileSystem remotely RPC calls the Namenode to create a new file in the namespace of the file system, which is not associated with any blocks at this time. During this process, the Namenode performs many verification tasks, such as whether there is a file with the same name, whether there are permissions, if the verification passes, it returns an FSDataOutputStream object. If the verification fails, an exception is thrown to the client.
When the client writes data, DFSOutputStream is decomposed into packets (data packets) and written to a data queue, which is consumed by DataStreamer.
DataStreamer is responsible for requesting the Namenode to allocate new blocks to store data nodes. These nodes store replicas of the same Block and form a pipeline. DataStreamer writes the packet to the first node of the pipeline. After the first node stores the packet, it forwards it to the next node, and the next node continues to pass it down.
DFSOutputStream also maintains an ack queue, waiting for confirmation messages from datanodes. After all datanodes on the pipeline confirm, the packet is removed from the ack queue.
After the data is written, the client closes the output stream. Flush all packets to the pipeline, and then wait for confirmation messages from datanodes. After all are confirmed, inform the Namenode that the file is complete. At this time, the Namenode already knows all the Block information of the file (because DataStreamer is requesting the Namenode to allocate blocks), and only needs to wait for the minimum replica number requirement to be reached, and then return a successful message to the client.
How does the Namenode determine which DataNode the replica is on?
The storage strategy of HDFS replicas is a trade-off between reliability, write bandwidth, and read bandwidth. The default strategy is as follows:
The first replica is placed on the machine where the client is located. If the machine is outside the cluster, a random one is selected (but it will try to choose a capacity that is not too slow or too busy).
The second replica is randomly placed on a rack different from the first replica.
The third replica is placed on the same rack as the second replica, but on a different node, and a random selection is made from the nodes that meet the conditions.
More replicas are randomly selected throughout the cluster, although too many replicas are avoided on the same rack as much as possible.
After the location of the replica is determined, when establishing the write pipeline, the network topology structure is considered. The following is a possible storage strategy:
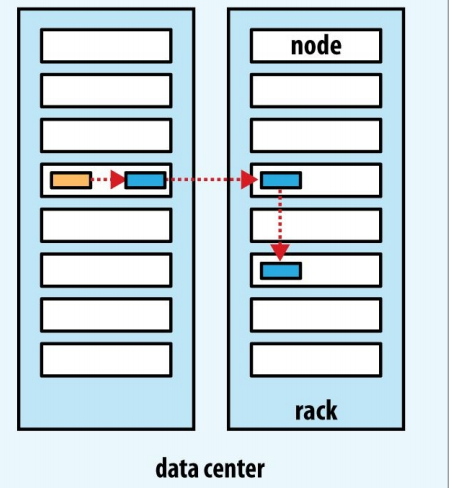
This selection balances reliability, read and write performance well.
Reliability: Blocks are distributed on two racks.
Write bandwidth: The write pipeline process only needs to cross one switch.
Read bandwidth: You can choose one of the two racks to read from.
Internal Features of HDFS
Data Redundancy
HDFS stores each file as a series of data blocks, with a default block size of 64MB (configurable).
For fault tolerance, all data blocks of a file have replicas (the replication factor is configurable).
HDFS files are written once and strictly limited to only one writing user at any time.
Replica Placement
HDFS clusters usually run on multiple racks, and communication between machines on different racks requires switches.
HDFS uses a rack-aware strategy to improve data reliability, availability, and network bandwidth utilization.
Rack failures are much less common than node failures, and this strategy can prevent data loss when an entire rack fails, improving data reliability and availability while ensuring performance.
Replica Selection
HDFS tries to use the replica closest to the program to satisfy user requests, reducing total bandwidth consumption and read latency.
HDFS architecture supports data balancing strategies.
Heartbeat Detection
The NameNode periodically receives heartbeats and block reports from each DataNode in the cluster. Receiving a heartbeat indicates that the DataNode is working properly.
The NameNode marks DataNodes that have not sent a heartbeat recently as dead and does not send them any new I/O requests.
The NameNode continuously checks for data blocks that need to be replicated and replicates them when necessary.
Data Integrity Check
Various reasons may cause the data block obtained from the DataNode to be corrupted.
HDFS client software implements checksum verification of HDFS file content.
If the checksum of the data block obtained by the DataNode is different from that in the hidden file corresponding to the data block, the client judges that the data block is corrupted and obtains a replica of the data block from another DataNode.
Simple Consistency Model, Stream Data Access
HDFS applications generally access files in a write-once, read-many mode.
Once a file is created, written, and closed, it does not need to be changed again.
This simplifies data consistency issues and makes high-throughput data access possible. Applications running on HDFS mainly focus on stream reading and batch processing, emphasizing high-throughput data access.
Client Cache
The request for the client to create a file does not immediately reach the NameNode. The HDFS client first caches the data to a local temporary file, and the write operation of the program is transparently redirected to this temporary file.
When the accumulated data in this temporary file exceeds the size of a block (64MB), the client contacts the NameNode.
If the NameNode crashes before the file is closed, the file will be lost.
If client caching is not used, network speed and congestion will have a significant impact on output.